Acquiring a new customer is more expensive than keeping an existing one
This is not just an oft-repeated marketing truism. Research cited in the Harvard Business Review shows that the cost of acquiring a new customer can be 5 to as much as 25 times the cost of retaining a customer, depending on the industry. And improving retention rates by just 5% can translate into as much as a 25% increase in profits. So how do we combat customer loss and increase retention? How can data analytics help us do so?
Customer churn (churn or attrition) is an inevitable phenomenon and it is impossible to eliminate it completely. Some customers, regardless of the measures taken against them, leave. For example, because they move out of the company’s area of operation or cease to be a target group and no longer need our product. The remainder, however, give up, opting for a competitor’s offer. These departures could have been prevented. If action had been taken. The right actions, at the right time. The keys are:
- Predicting the risk of customer departure with sufficient accuracy and in advance
- Understanding the factors that influence the risk of customer loss
The solution to both problems can be an anti-churn predictive model built using machine learning. Such a model is capable of predicting the risk of losing a particular customer. In doing so, it identifies the most important factors associated with an increase in this risk both generally for the entire customer base and individually for a single customer in his or her specific situation. Such predictive models can use any definition of “churn” and are applicable both to businesses where the departure of a customer is clearly marked in time (e.g., expiration/termination of a contract) and those where the customer simply stops returning and making further purchases.
The most important factors determining customer departure
As we mentioned, the predictive model helps identify the most important factors influencing the risk of customer churn. The charts below are from the actual predictive model built on one of Data Science Logic’s contractors. Only some of the variable names (including product category names) have been changed. It is worth noting that this is an industry characterized by a relatively low frequency of purchases (a few times a year on average) and high customer turnover.
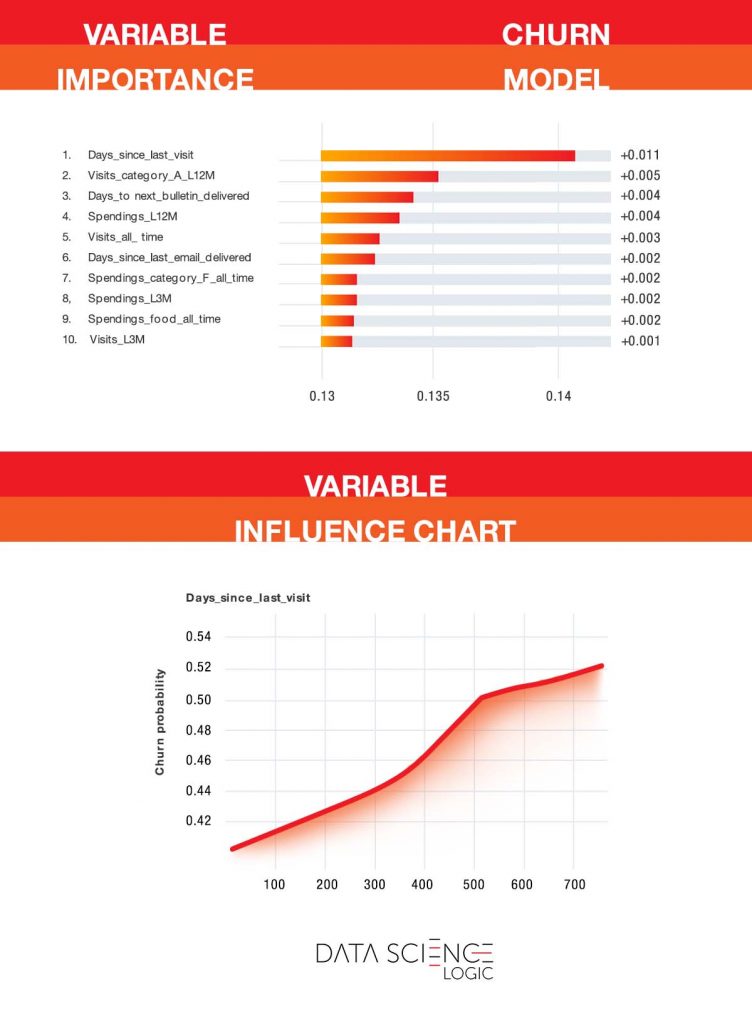
The chart at the top shows the customer characteristics that most explain the likelihood of leaving. As you can see, the key variable is the number of days since the last visit with a purchase. This is not surprising. The longer a customer has been gone, the less likely they are to return. However, the model allows you to pinpoint when the increase in risk is greatest and when you need to take decisive action. As you can see in the bottom graph, up to about 365 days the risk increases linearly. After more than one year of inactivity, the risk curve becomes steeper. This is the last moment to undertake an anti-churn campaign.
Also of interest is the second most important variable – the number of visits with a purchase of an “A” category product in the last 12 months. These products are exceptionally well regarded by customers and have a positive impact on customer satisfaction and retention.
In addition to general conclusions about the factors influencing the risk of losing customers, the model allows us to predict the probability of losing a particular person and to identify the specific characteristics that, in his case, increase or decrease this risk, as shown in the chart below. In his case, the risk is relatively low (35.5% compared to the baseline 49.6%). The risk is reduced by, among other things, the average value of the visit and the number of visits over the past year. However, the customer does not use the products of the aforementioned “A” category, which increases the risk of leaving. Encouraging them (e.g., through an appropriate campaign) to try products in this category would likely lower their risk of leaving even more.
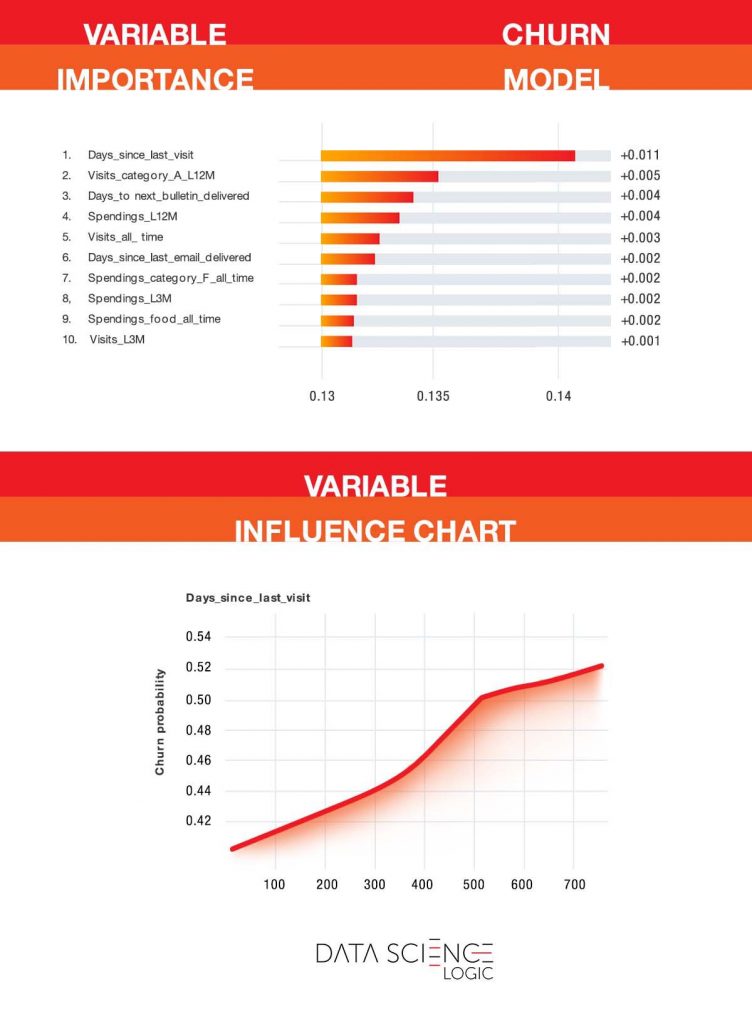
Dealing with customer migration is one of the most important challenges facing companies today, given how expensive it can be to acquire a new customer later on. With antichurn modeling, we will learn which customers are likely to leave and why, the signs of increasing risk of leaving, and how best to prevent them from leaving.