The modern consumer is constantly bombarded with messages. Competition for ever-shorter attention spans is bloody, and it’s all about leveraging every possible advantage. How can data science help in the successful battle to get a brand’s message to consumers? Learn the details of using machine learning in marketing communications.
What did marketing communications used to look like?
In the early years of the commercial Internet, when the use of e-mail in marketing communications was just beginning. The task was fairly simple. There was not yet much competition , the freshness effect worked, consumers were more likely to open and read messages. It can be said that just having an address base and executing any mailings was sufficient for success. It was sufficient to follow a simple model somewhat jokingly referred to as “Everything to Everyone Always” (EEA). The name reflects the tendency to send every prepared communication to the entire available addressee base.
The strategy was based on the assumption that every message sent increases the likelihood of a positive response to some degree. And that the cost of sending each additional message is close to zero. In the case of text messages, cost could of course be a limiting factor in the volume of messages sent. In a great many situations, however, this was dealt with simply by proportionally reducing the volume so as to exhaust, but not exceed, the allocated budget.
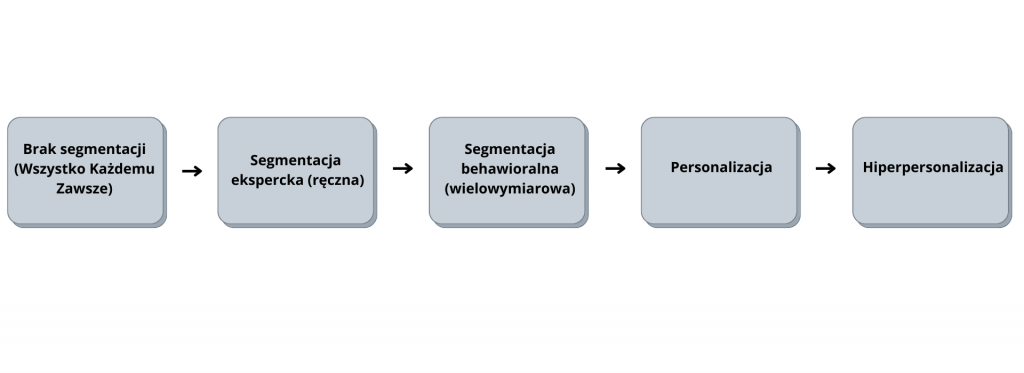
From no segmentation to hyper-personalization
The situation began to change with the increase in the number of brands using email and sms channels for communication. Consumers’ inboxes began to “burst at the seams.” The numbers of messages received exceeded the capacity of perception. Marketing communications in many cases began to be perceived as unwanted (“spam”). The widespread use of the PAC model mentioned earlier also contributed to this. Above all, the lack of customization of content to the specific consumer and too frequent mailings played a role. The seemingly zero cost of mailing (“at most, the customer won’t open it”) did not encourage investment of time and resources in targeting precision. What the calculations did not take into account, however, was that the consumer’s reaction to an overabundance of messages would be a gradual resistance to the message and a declining interest in opening the message.
The first step on the way out was to acknowledge that consumers at the base are not all the same. They have different needs and characteristics. Therefore, the content sent to them should be tailored accordingly. Segmentation of the base began. Manual segmentation based on expert knowledge and predefined segments prevailed. In this approach, the number of applicable consumer characteristics and segments was limited by human capabilities.
Increasing data
As the volume and scope of consumer data collected in databases increased, new opportunities opened up. The new data, combined with the growing computing power and sophistication of machine learning algorithms, made it possible to increase the number of features used in segmentation. Segmentation took on a behavioral character. With a large number of analyzed dimensions, it was able to take into account more complex aspects of consumer behavior and their relationship with the brand. The use of machine learning also made it possible to distinguish and analyze more segments. This, in turn, translated into greater consistency of the groups selected and allowed for better tailoring of communications to the audience.
The next step in the described evolution was the use of predictive models for more personalized tailoring of communications at the individual consumer level. Previously, segmentation allowed operating at the level of groups of consumers. Different segments may have received a different message at a different time, but all consumers belonging to a segment received the same thing. As the level of sophistication of segmentation increased, the number of segments could be larger and consumers assigned to them with greater accuracy. However, the level of generalization was still high, and the room for improvement in precision remained large. The use of predictive scoring models was a significant step forward. The model predicted the probability of each individual consumer’s interest in the subject of a given communication. It could have been, for example, a specific product, a product group, a promotional offer.
Machine learning
Based on consumer behavior data collected in the database, the algorithm learned patterns. Based on these, it was able to predict which of the palette of available messages would most positively influence a particular customer. This ensured that every customer in the database, regardless of the segment assigned to him, was able to receive the most appropriate content.
If, for any of the planned options, the model did not forecast sufficiently high consumer interest, the consumer could be excluded from a given mailing. This allowed a large-scale implementation of the principle that if we don’t have anything interesting to say for you at the moment, we’d better keep quiet. This allowed the consumer to feel that he was getting only news relevant to him. There is no shortage of examples of projects where such predictive models have been implemented. As a result, the perception of the recipients has changed from “stop sending me this spam” to “when will I get another newsletter”.
Using machine learning in marketing communications
The scoring models described, combined with a set of additional, even more advanced models, allow personalization to be taken another step further towards so-called hyperpersonalization. This is characterized by, among other things:
- fine-tuning the moment of dispatch. Each consumer can be assigned an ideal moment (in terms of time, day of the week, time since the previous mailing, time since the previous site visit, etc.).
- Selection of the best communication channel in conjunction with the ideal moment of dispatch.
- Selection of the best combination of channels used (a consumer may, for example, respond best to the combination of an email and a text message sent two days later).
- The ability to individualize content elements (e.g., choosing the right words in the subject line, selecting the best photo and other graphic elements.
- Dynamic adaptation of the message to the context of the consumer in real time. A different message and channel will be appropriate when the consumer is located in a shopping mall, and another when on the bus on the way to work.
Firstly, it is worth emphasizing that all the above-mentioned personalization takes place on the basis of behavioral patterns that models have “learned” by observing consumers and not on consumer declarations. A customer may declare that he would like to receive mailings on weekday mornings. In reality, mailings sent to him on Saturday evenings are most effective.
Secondly, the maximum power of the described solution comes from the synergy of the individual sub-models of the system. The optimal moment for a given consumer will vary from channel to channel. The optimal sequence and combination of channels may depend on the nature of the promotion being communicated. Finally, the optimal message text may depend on the channel and the location of the user.
Summary
Competition for consumers’ attention will increase as more channels of contact emerge, and it also increases as marketers become more aware of the tools that may be at their disposal. It’s worth asking ourselves at what stage of the described evolution our organization is at, and what we can do to take the next step to gain an additional advantage and not let the competition surpass us.