Today’s consumers are constantly inundated with messages from various brands. Many brands send multiple messages, through multiple channels. This makes it difficult to attract and keep the consumer’s attention for a long time. At the same time, it is easy for the consumer to become tired of the communication and pay less and less attention to it. Thus, it becomes more important than ever to choose the right content, to send the most tailored message to the consumer, and to limit messages that are not interesting and only increase the risk that the consumer will become insensitive to the message.
Predictive modeling is helping to solve the problem. Systems based on machine learning are able to predict consumer interest in a particular type of message or offer with a high degree of accuracy. The article uses a concrete and current example (from May 2023) to show how to apply the aforementioned tools in practice. Due to the highest standard of confidentiality, the numbers we will present will be scaled or shown as indexes. However, they will faithfully represent the observed differences and effects.
The problem with the traditional approach to email targeting and the need for change
The organization to which the example relates, like many others, for many years used a method of so-called “maximizing revenue” from its communications base through broad and frequent mailings. That is, in practice, information about an offer was sent to all consumers who had permission to communicate through a given channel. In a few cases, using expert criteria, the communicated base was narrowed down somewhat. However, this was based on simple criteria such as: has ever bought the promoted product before, has not bought product X in the last 6 months, is in a woman over 55, etc. The results were very good for a long time, and no one saw the need to change the process used. At some point, however, a slow decline in the email open rate (the so-called “open rate”) began to be observed. The downward trend began to be pronounced. Combined with the declining number of newly acquired consumers, this led the organization to wonder if it was possible to work better with the existing base. What can be done to reverse the trend of declining interest in the communications being sent?
The decision was made to test the integration of machine learning and predictive analytics into the process of selecting consumers for mailing campaigns. We prepared a predictive modeling system that generates “tailor-made” scoring models for each campaign. The general architecture of the system is shown in the diagram below.
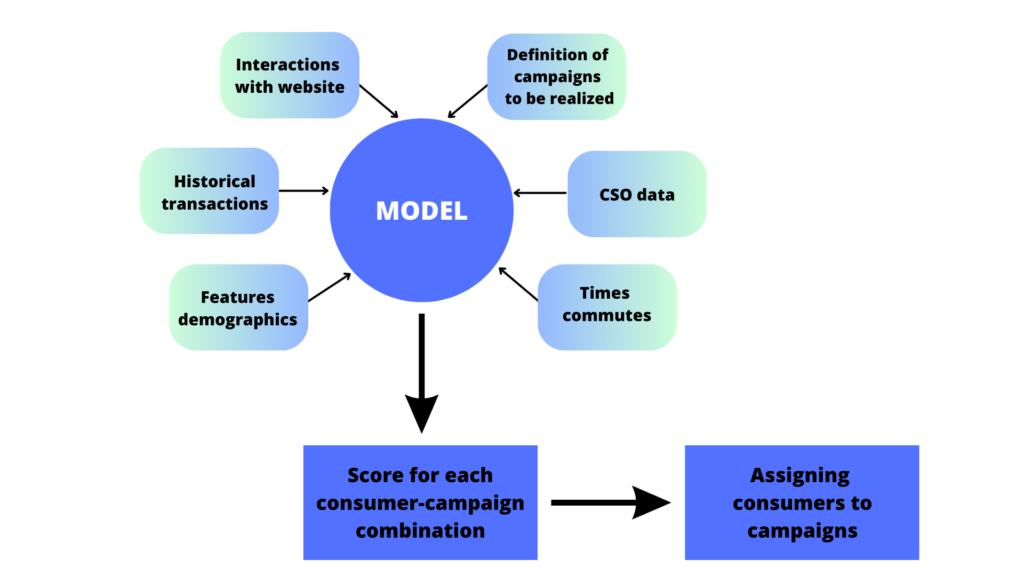
Use of predictive modeling in mail targeting
For the purpose of training the model, more than 100 variables from the areas listed in the diagram were used as input data. The model is built on the basis of advanced algorithms, able to cope with such a multitude of attributes and extract from them as much information as possible about the actual profile of the consumer. The final result is an estimate of the probability of interest in a given communication by each consumer. This is then used for the final selection of consumers for the campaign.
The results of the changes in the communication targeting process met (and even exceeded in some aspects) expectations. To prove the usefulness of the model, we conducted experiments. Half of the base was subjected to selection by the old way, while the other half was selected using the model’s prediction. It should be noted here that in both groups we used exactly the same emails – the same subject, exactly the same creation. Also, the timing of the mailing was the same. Therefore, none of these factors could have affected the results of the experiment. The only difference between the groups was the way consumers were selected.
Effects of using predictive modeling
In the group targeted with the model, it was possible to reduce the size of the communicated group by nearly 14 times – for every 100 communicated with traditional criteria, there are only 7 communicated according to the process based on the predictive model.
At the same time, such a small group generated similar (only about 2% lower) sales.
This was achieved by significantly higher (3 times) conversion in the group assigned to the campaign in the new way. And also a much (4 times) higher average receipt value in that group.
Narrowing the communicated group allowed to limit it to those really interested in the offer. This is evidenced by a much higher open rate (3.2x higher) and click to open rate (almost 2x higher). The click to open rate in this case is calculated as CTOR = LC/LO, where LC is the number of consumers who clicked on the link from the email, and LO is the number of consumers who opened the email. While the open rate is highly dependent on the subject line of the email, a higher CTOR indicates actual interest in the content and offer that is included in the email.
Targeting mailings based on predictive model – summary
By using an advanced data science tool in the form of a predictive model, it was possible to achieve:
- better matching of communications to consumer interests and needs
- a significant reduction in the number of communications in a given campaign with minimal damage to the sales result (just over 2%)
- reduction of communication “overload” – the consumer will receive communication less frequently but it will be better tailored in the new process
The exact impact of the model and the new targeting process on the trend of open and click-through rates of mailings, can only be studied over a longer period and requires at least several months of observation. However, the first recorded results look promising and give reason to expect a reversal of the clear negative trend seen in the months before the introduction of the scoring model.
Finally, it is worth noting that an advantage of the system is the openness of its architecture to new data sources. If new variables become available, they will be automatically incorporated into the model training process and used for prediction. Another important feature of the described solution is the model’s ability to update itself as new data arrives, including data on executed campaigns and their effectiveness. As a result, the model will automatically adapt to the changing needs and behavior of consumers and their reactions to the communications sent. This guarantees the usability of the system over the long term as well.